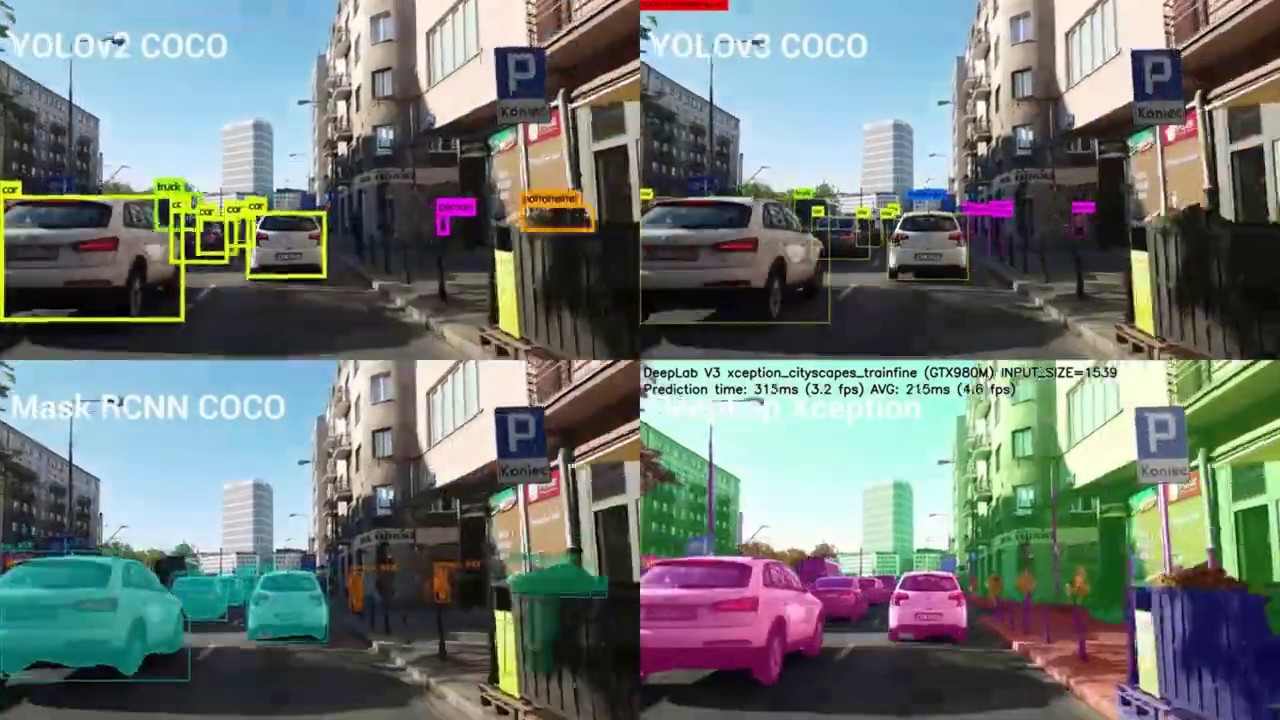
Student: Student: Leong Tian Poh Rech
Supervisor: Supervisor: Prof. Sye Loong Keoh (UGS)
Project Description:
Increasingly machine learning is used to automate many operations in our daily life, e.g., autonomous driving, spam email filtering, detection of network attacks, biometric authentication for automated access, object recognition, etc. However, this is based on the assumption that machine learning techniques were originally designed for stationary environments, where training and test data assumed to be trustworthy and obtained from the same distribution.
Recently, it has shown that it is possible to introduce adversary in the lifecycle of machine learning, where a malicious adversary can carefully manipulate the training data to exploit specific vulnerabilities of the learning algorithms to compromise the whole system. For example, In 2017 MIT researchers 3-D printed a toy turtle resulting in Google's object detection AI to classify it as a rifle. In another example, adversary tampered with the "stop" traffic sign, and resulting in the inability of the autonomous vehicle to recognize the traffic sign, and hence did not stop the vehicle.
This project aims to explore the severity of adversarial machine learning, by manipulating the neural network or generating adversarial input. The student should demonstrate the ability to compromise a machine learning system using a form of attack that is easy to use and robust to various conditions.
0 Comments